In the era of rapidly advancing technology, artificial intelligence (AI) projects have gained immense popularity and promise to revolutionize various industries. However, despite their potential, many AI initiatives end up stumbling on the road to success. We delve into the underlying reasons why AI projects often fail to live up to expectations, shedding light on crucial factors that contribute to their downfall. From data quality to inadequate expertise, ethical concerns to scalability issues, we uncover the challenges that can impede the progress of AI projects. Understanding these pitfalls is essential for organizations and individuals venturing into the world of AI to mitigate risks and increase the chances of achieving transformative outcomes.
So what are the crucial factors?
Implementing artificial intelligence technology holds great promise for businesses, but it is no secret that many AI initiatives do not achieve their intended outcomes. Understanding the reasons behind these failures is crucial for organizations looking to harness the full potential of AI.
Lack of clear objectives
One common factor that can lead to the failure of AI projects is a lack of clear objectives. Without well-defined goals and a precise understanding of what the AI project is expected to achieve, measuring success and progress becomes a daunting task. Unclear objectives can result in a lack of focus and direction, causing the project to drift or lose momentum. It is imperative for organizations to establish specific and measurable objectives to guide the AI project's development and evaluation.
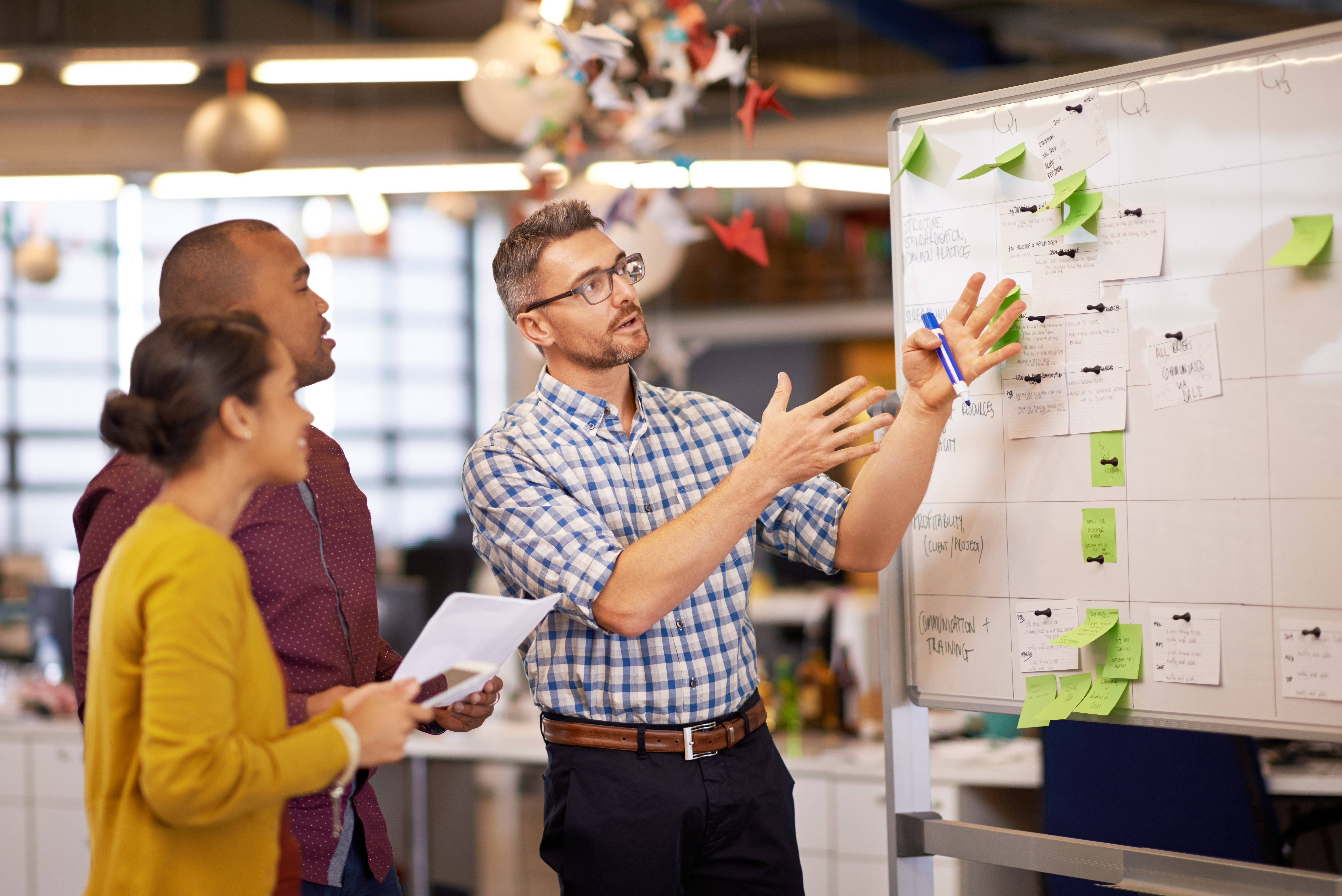
Insufficient data quality and quantity
Another significant challenge is the insufficient quality or quantity of data. AI algorithms heavily rely on data for training and making accurate predictions. If the data used for training is of poor quality, incomplete, or biased, it can significantly impact the performance of the AI system. Inadequate data quantity can also limit the effectiveness of AI algorithms, as they may not have enough examples to learn from. Organizations must ensure they have access to high-quality, diverse, and sufficient data to achieve optimal results.
AI initiatives require robust data infrastructure and efficient data governance practices. Inadequate data storage, inconsistent formats, and lack of data access can hinder AI implementation and performance.
Inadequate expertise and resources
Successful AI implementation demands a skilled team with expertise in data science, machine learning, and domain knowledge. Lack of appropriate talent, training, and resources can hamper progress and limit the effectiveness of AI initiatives.
Effective project management is critical for the success of AI initiatives. Poor planning, inadequate resource allocation, lack of communication and coordination among team members, and unrealistic timelines can all contribute to project failure. To mitigate this risk, organizations should adopt robust project management practices, ensuring clear communication channels, realistic timelines, and appropriate resource allocation to foster efficient project execution.
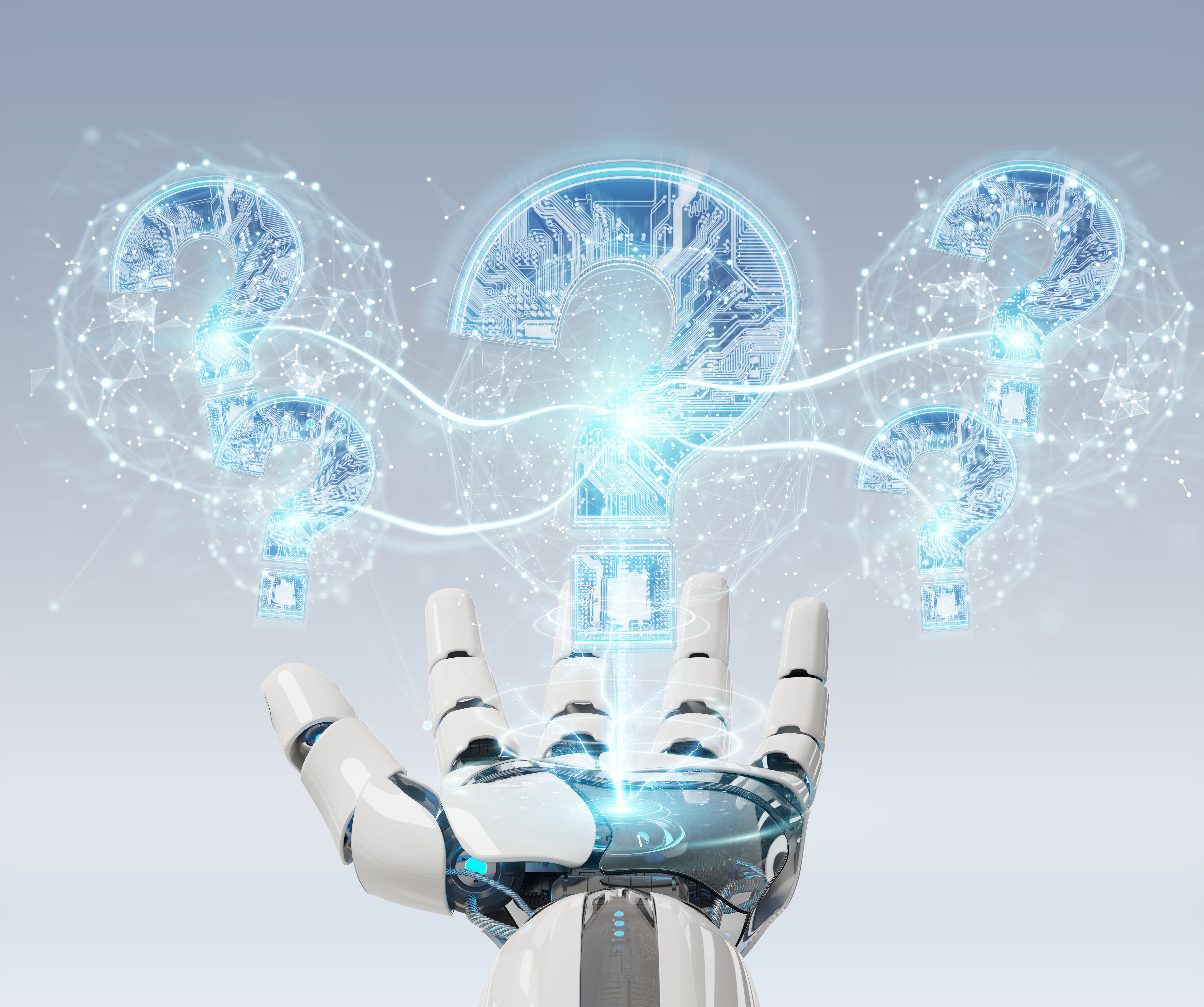
Ethic and law
Ethical and legal challenges pose significant risks to AI projects. AI systems can raise concerns such as bias, privacy violations, and lack of transparency. Failing to address these issues adequately can lead to public backlash, regulatory challenges, and ultimately project failure. Organizations must prioritize ethical considerations and ensure compliance with relevant legal frameworks to build trust, minimize risks, and safeguard the reputation of the project and the organization.
Unrealistic expectations
Sometimes, overhyped expectations surrounding AI can lead to disappointment. AI is not a magical solution that can instantly solve all problems. It is crucial to have realistic expectations and understand the limitations of AI technologies.
Finally, the lack of user adoption and acceptance can undermine the effectiveness of AI solutions. Regardless of the system's technical capabilities, AI solutions rely on end-users embracing and utilizing them. If users do not perceive value or trust in the AI system, they may resist adoption, rendering the project ineffective.
Implementing AI often requires significant organizational changes and a shift in mindset. Resistance to change, lack of buy-in from key stakeholders, and cultural barriers can impede successful adoption and integration of AI systems.
High costs and long development times
Cost and return on investment (ROI) considerations also play a significant role in AI project outcomes. AI projects can be resource-intensive, requiring substantial investments in data infrastructure, computing resources, and talent. Organizations must have a clear understanding of the expected ROI, ensuring that the costs incurred align with the anticipated benefits. Proper cost-benefit analysis and effective resource allocation strategies are crucial to ensure the project's viability and success. Organizations invest money in full-fledged solutions instead of testing basic/prototype versions with the target users.
To overcome these challenges and increase the likelihood of AI success, organizations must approach AI implementation with careful planning, robust data strategies, investment in talent, and a clear understanding of the ethical and legal implications. By addressing these factors proactively, businesses can harness the true potential of AI and drive transformative outcomes.